データ分析に関する備忘録。主にR言語を使ったデータの前処理や統計、機械学習などの方法を記録。ビッククエリとトレジャーデータがお気に入り。オフラインとオンラインの連携が最近のマイブーム。
注目の投稿
【kepler.gl】コロナ対策による人流の変化も地図上に可視化(各種メディアで報道)
kepler.glのサイト画面 kepler.glを使ってコロナ対策の効果を分析したところ、テレビ、新聞、ネットのメディアから問い合わせや報道依頼が殺到。今も、土日返上で都内や全国の人流変化を分析しています。この記事では人流変化の可視化に便利なkepler.glにつ...
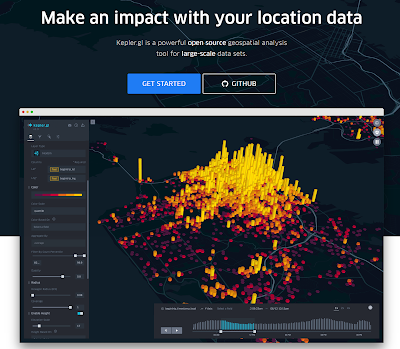
2016年5月31日火曜日
最終出社日
今日は早めに会社を出て明治神宮で感謝のご報告
いつものように会社に出社
出て行く会社、新たに入る会社
出て行く会社には今後のご発展をお祈り申し上げます
新たに入る会社には異次元の成長を約束します!
うぉぉ~、がんばるぞぉぉぉ~~~~~~~~!!
いつものように会社に出社
出て行く会社、新たに入る会社
出て行く会社には今後のご発展をお祈り申し上げます
新たに入る会社には異次元の成長を約束します!
うぉぉ~、がんばるぞぉぉぉ~~~~~~~~!!
2016年5月30日月曜日
2016年5月27日金曜日
【論文紹介】Emergence and Stability of Key Currency in Aritificial Internatonal Trade
ちょっと前、基軸通貨が話題になった時期がありましたよね
リーマンショックの際、ドルに変わる新たな基軸通貨が必要とか
より安定的な基軸通貨が必要とか
少し昔(2005年)の論文ですが、シミュレーションを用いて基軸通貨の創発・安定メカニズムを議論している研究があったので紹介、というかメモ書きです
タイトル:
Emergence and Stability of Key Currency in Aritificial Internatonal Trade
内容:
目的は、国家の経済力が等しい場合における基軸通貨の創発・安定メカニズムの解明。方法は、「通貨の信用度」の概念を導入した人工国際市場モデルによるシミュレーション。モデルの前提は、発展した金融システムのない単純な貿易・金融市場。明らかにしたのは、以下の3点。
論文:
Yamashita, T., K. Kurumatani, Y. Sasaki, H. Kawamura, and A. Ohuchi (2005). “Emergence and Stability of Key Currency in Articial International Trade”. New Generation Computing, 23(1) pp.13-22.
論文の入手先:
http://www.springerlink.com/content/kg21005470n66rg0/
リーマンショックの際、ドルに変わる新たな基軸通貨が必要とか
より安定的な基軸通貨が必要とか
少し昔(2005年)の論文ですが、シミュレーションを用いて基軸通貨の創発・安定メカニズムを議論している研究があったので紹介、というかメモ書きです
タイトル:
Emergence and Stability of Key Currency in Aritificial Internatonal Trade
内容:
目的は、国家の経済力が等しい場合における基軸通貨の創発・安定メカニズムの解明。方法は、「通貨の信用度」の概念を導入した人工国際市場モデルによるシミュレーション。モデルの前提は、発展した金融システムのない単純な貿易・金融市場。明らかにしたのは、以下の3点。
- 基軸通貨が国家の経済力が対称的な状況で創発
- 外国為替トレーダーの働きによって市場の安定化が助長
- 外国為替トレーダーの働きによって各国の生産特化が促進
論文:
Yamashita, T., K. Kurumatani, Y. Sasaki, H. Kawamura, and A. Ohuchi (2005). “Emergence and Stability of Key Currency in Articial International Trade”. New Generation Computing, 23(1) pp.13-22.
論文の入手先:
http://www.springerlink.com/content/kg21005470n66rg0/
ラベル:
key currency
,
シミュレーション
,
基軸通貨
,
研究
,
論文紹介
2016年5月26日木曜日
2016年5月25日水曜日
【THEO運用状況】_2016年5年24日
THEO(テオ)ってやつが面白そうなので試しに始めてみました
今のところマイナス…
まあ、気長に待ちましょう。。。
THEO紹介ページ
https://theo.blue/main?utm_expid=116960401-4.zq7wLmzdTfqrCtpjq65mcA.1&utm_referrer=https%3A%2F%2Ftheo.blue%2Fmain
今のところマイナス…
まあ、気長に待ちましょう。。。
THEO紹介ページ
https://theo.blue/main?utm_expid=116960401-4.zq7wLmzdTfqrCtpjq65mcA.1&utm_referrer=https%3A%2F%2Ftheo.blue%2Fmain
2016年5月24日火曜日
【転職】データサイエンティスト → 社長室
某会社のデータサイエンティストから某会社の社長室に転職することになった
うぉーーーー、がんばるぞぉぉぉぉぉぉぉーーーーーーー!!!
(転職)できる、できないではない
(転職)するか、しないかだ キリ!
うぉーーーー、がんばるぞぉぉぉぉぉぉぉーーーーーーー!!!
(転職)できる、できないではない
(転職)するか、しないかだ キリ!
ラベル:
データサイエンティスト
,
社長室
,
転職
【メモ】最低限KPIでいるもの
UU
課金階級別PU
課金階級別課金額
最高階級のARPPU
あと、新規のKPIがめっちゃ重要
これがないと集客が悪いのかゲームの施策等が悪いのかが分からない
新規UU
新規課金率
課金階級別PU
課金新規階級別課金額
新規最高階級のARPPU
課金階級別PU
課金階級別課金額
最高階級のARPPU
あと、新規のKPIがめっちゃ重要
これがないと集客が悪いのかゲームの施策等が悪いのかが分からない
新規UU
新規課金率
課金階級別PU
課金新規階級別課金額
新規最高階級のARPPU
【研究】金融危機は未然に予測し、防止することが可能:経済界を大きく変える「ドラゴンキング理論」
金融危機は未然に予測し、防止することが可能:経済界を大きく変える「ドラゴンキング理論」
http://u-note.me/note/47499423
あ、ドラゴンキング!5年くらい前に論文読んだなー。なつかしすぎる。
まとめ
ドラゴンキングとは?
---論文概要---
分布の中心から極端に乖離した値は,通常,外れ値として処理される.しかし,その分布がべき乗則に従うものであれば,その外れ値は,分布の特性を示すテールとなってあらわれ,正規分布での予想を遥かに超える高い頻度で発生する.本論文では,以上のようなベキ分布におけるテールに注目し,稀に起きかつ甚大な被害をもたらす金融危機や大災害の予測可能性について議論し,同時に,それらを予測するための新たな概念としてドラゴンキングを提唱する.ドラゴンキングを分析することで危機の前兆を捉えることが可能になる.
http://u-note.me/note/47499423
あ、ドラゴンキング!5年くらい前に論文読んだなー。なつかしすぎる。
まとめ
ドラゴンキングとは?
- 通常、外れ値として処理される極端な事象
- 外れ値ではなく特殊なメカニズムを持つ
- メカニズム持つため予測・制御可能
- ドラゴンキングの論文はこちら
---論文概要---
分布の中心から極端に乖離した値は,通常,外れ値として処理される.しかし,その分布がべき乗則に従うものであれば,その外れ値は,分布の特性を示すテールとなってあらわれ,正規分布での予想を遥かに超える高い頻度で発生する.本論文では,以上のようなベキ分布におけるテールに注目し,稀に起きかつ甚大な被害をもたらす金融危機や大災害の予測可能性について議論し,同時に,それらを予測するための新たな概念としてドラゴンキングを提唱する.ドラゴンキングを分析することで危機の前兆を捉えることが可能になる.
【学会】Workshop on Communicating Intentions in Human-Robot Interaction
Workshop on Communicating Intentions in Human-Robot Interaction のお知らせ
August 31, 2016
Columbia University, New York
http://www.intentions.xyz/roman-2016-workshop/
Submission deadline: June 24, 2016 (23:59 PST)
===================================================
Call for papers
Workshop on Communicating Intentions in Human-Robot Interaction
August 31, 2016
in conjunction with
IEEE International Symposium on Robot and Human
Interactive Communication (RO-MAN 2016)
August 26-31, 2016
Columbia University, New York
===================================================
---[Dates]---
Submission deadline: June 24, 2016 (23:59 PST)
Notification of acceptance: July 8, 2016
Workshop date: August 31, 2016
---[Background and motivation]---
Research in the cognitive sciences, in particular social neuroscience, has in recent
years made substantial progress in elucidating the mechanisms underlying the
recognition and communication of intentions in natural human-human social interactions
and in developing computational models of these mechanisms. However, there is
much less research on the mechanisms underlying the human interpretation of
the behavior of artifacts, such as robots or automated vehicles, and the attribution
of intentions to such systems.
Furthermore, robots’ recognition of human intentions is arguably a prerequisite
for pro-social behavior, and necessary to engage in, for instance, instrumental
helping or mutual collaboration. To develop robots that can interact naturally
and effectively with people therefore requires the creation of systems that can
perceive and comprehend intentions in other agents.
For research on social interactions between humans and robots/agents in general,
and mutual recognition/communication of intentions in particular, it is therefore
important to be clear about the theoretical framework and inherent assumptions
underlying technological implementations. This also has ramifications for the evaluation
of the quality of human-robot interactions.
Overall, the role of intentions in human-robot interaction very much remains an active
and growing research area in which further development is necessary, and the purpose
of this workshop is to advance the state of the art in that respect. The intended audience
consists of researchers from robotics, AI and the cognitive sciences. The focus is on
interdisciplinary interaction.
---[Workshop Content]---
The workshop will be centered around three main activities:
(i) keynote presentations to highlight the overall state of the art;
(ii) paper presentations that deal with specific aspects of the work carried out
by workshop participants;
(iii) a round-table discussion that will allow all participants to contribute their thoughts
on the open and most pressing research challenges.
---[Scope]---
Suitable topics for the workshop address intention communication in Human-Robot Interaction;
for instance:
* mechanisms of intention communication in Human-Robot interaction;
* machine recognition of human intentions;
* human recognition/attribution of robot intentions;
* implications for the evaluation of HRI.
We particularly encourage papers that consider mutual
recognition/communication of intentions (i.e. that consider
both human recognition of robot intentions and robot recognition
of human intentions in given application contexts), but will
also consider papers that deal with uni-directional intention
recognition/communication. Papers can be pure position
papers, or can substantiate their message with empirical work.
Papers will be peer-reviewed and we emphasize that papers must make an
interesting, relevant, and novel contribution (whether theoretical or
empirical) to the state of the art.
---[Submission Instructions]---
We expect papers to be 4 - 8 pages using the IEEE conference templates
(available at
http://www.ieee.org/conferences_events/conferences/publishing/templates.html).
Please e-mail your paper to tom.ziemke@his.se <mailto:tom.ziemke@his.se>, serge.thill@his.se <mailto:serge.thill@his.se> and
alberto.montebelli@his.se <mailto:alberto.montebelli@his.se> by the submission deadline.
---[Publication]---
Preliminary proceedings will be published in the "Skövde University
Studies in Informatics" series (ISSN 1653-2325). Authors have the right
to opt out of these proceedings. We will further organize a Frontiers
(in Neurorobotics) research topic as a venue for extended papers on the
themes of the workshop.
---[Website]---
News and updates will be available at:
http://www.intentions.xyz/roman-2016-workshop/
---[Organisers]—
Tom Ziemke, Linköping University & University of Skövde, Sweden
Serge Thill, University of Skövde, Sweden
Alberto Montebelli, University of Skövde, Sweden
2016年5月23日月曜日
【ひと休み】100年にひとりの「笑点」司会者!!
最近はやりの
「○○年にひとりの××は」
本当に統計を取ったらどんな値になるんだろ
春風亭昇太は100年にひとりの「笑点」司会者になるか
http://www.excite.co.jp/News/reviewmov/20160523/E1463965826166.html?_p=2
「○○年にひとりの××は」
本当に統計を取ったらどんな値になるんだろ
春風亭昇太は100年にひとりの「笑点」司会者になるか
http://www.excite.co.jp/News/reviewmov/20160523/E1463965826166.html?_p=2
ラベル:
ひと休み
【行動経済学】損失は利得の2倍以上のインパクトがある
損失回避まとめ
損失回避性が選択行動にバイアスをもたらす例
コメント
よくスーパーがやってる スタンプとかポイントとかは損失回避をうまく利用してるってことか
購入する → ポイント溜まる → 無駄にしたくないからまた同じお店で購入
参照
人はなぜ変われないのかーー
- 損失回避:人は得るよりも失うことの心理的影響が強い
- 心理実験:数値としては2~2.5倍の重みがある
損失回避性が選択行動にバイアスをもたらす例
- サンクコスト効果:損失を回避したいがために合理的な判断ができなくなる
- 現状維持バイアス:保持する高く評価して手放さなくなる
コメント
よくスーパーがやってる スタンプとかポイントとかは損失回避をうまく利用してるってことか
購入する → ポイント溜まる → 無駄にしたくないからまた同じお店で購入
参照
人はなぜ変われないのかーー
「損失を回避する」本能が行動に影響
https://www.teamspirit.co.jp/catalyst/column/change-01.html【R】 任意の月次新規集客数を指定して将来のユーザ動態をシミュレートする
下記、Rのコード(備忘録用)
#内容:任意の月次新規集客数から将来のユーザ動態をシミュレートする
#必要に応じてパッケージをインストール 下記一例
#install.packages("rpart")
#install.packages("rpart.plot")
#install.packages("partykit")
#install.packages("rattle")
#install.packages("ggplot2")
#install.packages("sna")
library(data.table) #データ読み込み fread
library(dplyr) #SQLのような集計処理 group_by, summarise
library(rpart) #決定木
library(rattle) # 描画用ライブラリ
library(rpart.plot) # 描画用ライブラリ
library(partykit)
#ネットワーク作図用
library(nnet)
library(MASS)
library(reshape2)
library(ggplot2)
library(sna)
#月次課金額を計算する際の基準とする
set.now_firstday <- as.Date("2016-02-01")
set.now_endofmonth <- as.Date("2016-03-01")
newusers <- 50000 #新規ユーザ数を任意に設定
ActZrate <- 0.5 #前月act(A~NP)のZの割合(前月ログインユーザの翌月ログイン無率)
#3か月分のdauをそれぞれ読み込む
df.dau_before <- fread("./dau_count_2016-01-01_to_2016-01-31_Total.csv")
df.dau_before <- data.frame(df.dau_before)
df.dau_before <- df.dau_before %>%
group_by(user_id) %>%
summarise(start_time = min(start_time))
df.dau_now <- fread("./dau_count_2016-02-01_to_2016-02-29_Total.csv")
df.dau_now <- data.frame(df.dau_now)
df.dau_now <- df.dau_now %>%
group_by(user_id) %>%
summarise(start_time = min(start_time))
df.dau_next <- fread("./dau_count_2016-03-01_to_2016-03-31_Total.csv")
df.dau_next <- data.frame(df.dau_next)
df.dau_next <- df.dau_next %>%
group_by(user_id) %>%
summarise(start_time = min(start_time))
#2か月分の課金額を読み込む
df.purchase_all <- fread("./purchase_20160201_20160331.csv")
df.purchase_all <- data.frame(df.purchase_all)
#null
df.purchase_all[is.na(df.purchase_all)] <- 0
#登録日から現在までの利用期間を計算
set.basetime <- set.now_endofmonth
df.dau_before$playtime <- set.basetime - as.Date(df.dau_before$start_time)
df.dau_now$playtime <- set.basetime - as.Date(df.dau_now$start_time)
#月次課金額をユーザ別に集計
df.purchase_now <- df.purchase_all %>%
filter(paid_at < set.now_endofmonth) %>%
group_by(user_id) %>%
summarize(total = sum(price))
df.purchase_next <- df.purchase_all %>%
filter(paid_at >= set.now_endofmonth) %>%
group_by(user_id) %>%
summarize(total = sum(price))
#課金額に応じてクラス分けする
df.purchase_now$class <- "G"
df.purchase_now[df.purchase_now$total <= 1000,]$class <- "F"
df.purchase_now[df.purchase_now$total > 1000 & df.purchase_now$total <= 3000,]$class <- "E"
df.purchase_now[df.purchase_now$total > 3000 & df.purchase_now$total <= 10000,]$class <- "D"
df.purchase_now[df.purchase_now$total > 10000 & df.purchase_now$total <= 30000,]$class <- "C"
df.purchase_now[df.purchase_now$total > 30000 & df.purchase_now$total <= 100000,]$class <- "B"
df.purchase_now[df.purchase_now$total > 100000,]$class <- "A"
df.purchase_next$class <- "G"
df.purchase_next[df.purchase_next$total <= 1000,]$class <- "F"
df.purchase_next[df.purchase_next$total > 1000 & df.purchase_next$total <= 3000,]$class <- "E"
df.purchase_next[df.purchase_next$total > 3000 & df.purchase_next$total <= 10000,]$class <- "D"
df.purchase_next[df.purchase_next$total > 10000 & df.purchase_next$total <= 30000,]$class <- "C"
df.purchase_next[df.purchase_next$total > 30000 & df.purchase_next$total <= 100000,]$class <- "B"
df.purchase_next[df.purchase_next$total > 100000,]$class <- "A"
#新規登録ユーザIDを特定
df.newuserid <- df.dau_now %>%
filter(start_time >= set.now_firstday)
#必要なカラムのみ抽出
#dau
df.dau_before <- df.dau_before %>%
dplyr::select(user_id, playtime)
df.dau_now <- df.dau_now %>%
dplyr::select(user_id, playtime)
df.dau_next <- df.dau_next %>%
dplyr::select(user_id)
df.purchase_now <- df.purchase_now %>%
dplyr::select(user_id,class)
df.purchase_next <- df.purchase_next %>%
dplyr::select(user_id,class)
#既存ユーザ属性特定
#join to dau
df.all_now <- left_join(df.dau_now,df.purchase_now,by = "user_id")
df.all_now[is.na(df.all_now)] <- "NP"
#離脱ユーザの特定と新規除外
#z:before > now
df.all_now_old <- left_join(df.dau_before,df.all_now, by = "user_id")
df.all_now_old[is.na(df.all_now_old)] <- "Z"
#np:now > next
df.all_next <- left_join(df.dau_next,df.purchase_next,by = "user_id")
df.all_next[is.na(df.all_next)] <- "NP"
#z:now > next
df.all_now_old <- left_join(df.all_now_old, df.all_next, by = "user_id")
df.all_now_old[is.na(df.all_now_old)] <- "Z"
#rename and select
df.all_old <- df.all_now_old %>%
dplyr::select(user_id, playtime.x, class.x, class.y)
names(df.all_old) <- c("user_id","playtime","class_now","class_next")
#新規ユーザ属性特定
df.dau_now_new <- left_join(df.newuserid, df.dau_now, by = "user_id")
df.all_now_new <- left_join(df.dau_now_new, df.purchase_now, by = "user_id")
df.all_now_new[is.na(df.all_now_new)] <- "NP"
#z:now > next
df.all_now_new <- left_join(df.all_now_new, df.all_next, by = "user_id")
df.all_now_new[is.na(df.all_now_new)] <- "Z"
#rename and select
df.all_new <- df.all_now_new %>%
dplyr::select(user_id, playtime.x, class.x, class.y)
names(df.all_new) <- c("user_id","playtime","class_now","class_next")
#新規をrbind
df.all <- rbind(df.all_new, df.all_old)
#決定木モデル構築
#playtimは変数として使うとシミュレーションが困難となるため使用しない
tree <- rpart(class_next ~ class_now,data = df.all,control=rpart.control(minsplit=1, cp=0.0001))
dev.off() #エラー回避→ Error in .Call.graphics(C_palette2, .Call(C_palette2, NULL)) :invalid graphics state
plot(as.party(tree))
plotcp(tree)
#遷移確率
class_now <- df.all$class_now
df.test <- data.frame(class_now)
df.pred <- predict(tree,df.test)
df.pred <- data.frame(df.pred)
df.pred <- cbind(df.test,df.pred) #横に連結
#write.csv(df.pred,"./output/pred.csv")
#確率
df.pred_Pv <- melt(df.pred, id.vars = c("class_now"),variable.name = "class_next", na.rm = TRUE)
df.pred_Pv <- df.pred_Pv %>%
group_by(class_now,class_next) %>%
summarise(Pv = mean(value))
write.csv(df.pred_Pv,"./output/pred_Pv.csv")
#初期化 ↓
#initial data
df.initialdata <- df.all_now
df.initialdata <- df.initialdata %>%
group_by(playtime, class) %>%
summarise(uu = length(user_id))
names(df.initialdata) <- c("playtime","class_now","uu")
#sum = a~npの合計
anp_before <- sum(df.initialdata$uu)
#newuser
df.newuser_PayPv <- left_join(df.dau_now,df.purchase_now,by = "user_id")
df.newuser_PayPv <- df.newuser_PayPv %>%
filter(playtime <= 30) #1ヶ月は30日とする
df.newuser_PayPv[is.na(df.newuser_PayPv)] <- "NP"
#newuser Pv
df.newuser_PayPv <- df.newuser_PayPv %>%
group_by(class) %>%
summarize(uu = length(user_id))
df.newuser_PayPv$Pv <- df.newuser_PayPv$uu / sum(df.newuser_PayPv$uu)
#設定
df.newuser_PayPv$uu <- df.newuser_PayPv$Pv * newusers
df.newuser_PayPv$playtime <- 1
df.newuser_PayPv <- df.newuser_PayPv %>%
group_by(playtime, class) %>%
summarise(uu = sum(uu))
names(df.newuser_PayPv) <- c("playtime","class_now","uu")
df.result <- df.initialdata
df.result$turn <- 0
#simulation 最初の予測
#変数データを用意 playtime class_now
df.sim <- left_join(df.initialdata, df.pred_Pv, by = "class_now")
df.sim$uu_next <- df.sim$uu * df.sim$Pv
df.sim <- df.sim %>%
group_by(playtime, class_next) %>%
summarise(uu = sum(uu_next))
names(df.sim) <- c("playtime","class_now","uu")
df.sim$playtime <- df.sim$playtime + 30 #1ヶ月は30日とする
#次の新規挿入
df.sim <- rbind(df.newuser_PayPv,df.sim)
#前月actZrate
df.sim[df.sim$class_now == "Z",]$uu <- df.sim[df.sim$class_now == "Z",]$uu * ActZrate
#初期値に代入
df.initialdata <- df.sim
df.result_test <- df.initialdata
df.result_test$turn <- 1
df.result <- rbind(df.result, df.result_test)
#simulation 2回目以降の予測
#初期化
#予測
for(i in 2:30){ #30ヵ月後までシミュレート(任意)
#変数データを用意 playtime class_now
df.sim <- left_join(df.initialdata, df.pred_Pv, by = "class_now")
df.sim$uu_next <- df.sim$uu * df.sim$Pv
df.sim <- df.sim %>%
group_by(playtime, class_next) %>%
summarise(uu = sum(uu_next))
names(df.sim) <- c("playtime","class_now","uu")
df.sim$playtime <- df.sim$playtime + 30 #1ヶ月は30日とする
#前月actZrate
df.sim[df.sim$class_now == "Z",]$uu <- df.sim[df.sim$class_now == "Z",]$uu * ActZrate
#次の新規挿入
df.sim <- rbind(df.newuser_PayPv,df.sim)
#初期値に代入
df.initialdata <- df.sim
df.result_test <- df.initialdata
df.result_test$turn <- i
df.result <- rbind(df.result, df.result_test)
}
#結果出力
write.csv(df.result,"./output/result.csv")
#遷移確率のネットワーク図
df.Pv_netwaork <- acast(df.pred_Pv, class_now ~ class_next, max, value.var = "Pv")
gplot(df.Pv_netwaork,mode = "circle",edge.lwd = df.Pv_netwaork*15, edge.col = "#c8c8cb", displaylabels = TRUE)
#内容:任意の月次新規集客数から将来のユーザ動態をシミュレートする
#必要に応じてパッケージをインストール 下記一例
#install.packages("rpart")
#install.packages("rpart.plot")
#install.packages("partykit")
#install.packages("rattle")
#install.packages("ggplot2")
#install.packages("sna")
library(data.table) #データ読み込み fread
library(dplyr) #SQLのような集計処理 group_by, summarise
library(rpart) #決定木
library(rattle) # 描画用ライブラリ
library(rpart.plot) # 描画用ライブラリ
library(partykit)
#ネットワーク作図用
library(nnet)
library(MASS)
library(reshape2)
library(ggplot2)
library(sna)
#月次課金額を計算する際の基準とする
set.now_firstday <- as.Date("2016-02-01")
set.now_endofmonth <- as.Date("2016-03-01")
newusers <- 50000 #新規ユーザ数を任意に設定
ActZrate <- 0.5 #前月act(A~NP)のZの割合(前月ログインユーザの翌月ログイン無率)
#3か月分のdauをそれぞれ読み込む
df.dau_before <- fread("./dau_count_2016-01-01_to_2016-01-31_Total.csv")
df.dau_before <- data.frame(df.dau_before)
df.dau_before <- df.dau_before %>%
group_by(user_id) %>%
summarise(start_time = min(start_time))
df.dau_now <- fread("./dau_count_2016-02-01_to_2016-02-29_Total.csv")
df.dau_now <- data.frame(df.dau_now)
df.dau_now <- df.dau_now %>%
group_by(user_id) %>%
summarise(start_time = min(start_time))
df.dau_next <- fread("./dau_count_2016-03-01_to_2016-03-31_Total.csv")
df.dau_next <- data.frame(df.dau_next)
df.dau_next <- df.dau_next %>%
group_by(user_id) %>%
summarise(start_time = min(start_time))
#2か月分の課金額を読み込む
df.purchase_all <- fread("./purchase_20160201_20160331.csv")
df.purchase_all <- data.frame(df.purchase_all)
#null
df.purchase_all[is.na(df.purchase_all)] <- 0
#登録日から現在までの利用期間を計算
set.basetime <- set.now_endofmonth
df.dau_before$playtime <- set.basetime - as.Date(df.dau_before$start_time)
df.dau_now$playtime <- set.basetime - as.Date(df.dau_now$start_time)
#月次課金額をユーザ別に集計
df.purchase_now <- df.purchase_all %>%
filter(paid_at < set.now_endofmonth) %>%
group_by(user_id) %>%
summarize(total = sum(price))
df.purchase_next <- df.purchase_all %>%
filter(paid_at >= set.now_endofmonth) %>%
group_by(user_id) %>%
summarize(total = sum(price))
#課金額に応じてクラス分けする
df.purchase_now$class <- "G"
df.purchase_now[df.purchase_now$total <= 1000,]$class <- "F"
df.purchase_now[df.purchase_now$total > 1000 & df.purchase_now$total <= 3000,]$class <- "E"
df.purchase_now[df.purchase_now$total > 3000 & df.purchase_now$total <= 10000,]$class <- "D"
df.purchase_now[df.purchase_now$total > 10000 & df.purchase_now$total <= 30000,]$class <- "C"
df.purchase_now[df.purchase_now$total > 30000 & df.purchase_now$total <= 100000,]$class <- "B"
df.purchase_now[df.purchase_now$total > 100000,]$class <- "A"
df.purchase_next$class <- "G"
df.purchase_next[df.purchase_next$total <= 1000,]$class <- "F"
df.purchase_next[df.purchase_next$total > 1000 & df.purchase_next$total <= 3000,]$class <- "E"
df.purchase_next[df.purchase_next$total > 3000 & df.purchase_next$total <= 10000,]$class <- "D"
df.purchase_next[df.purchase_next$total > 10000 & df.purchase_next$total <= 30000,]$class <- "C"
df.purchase_next[df.purchase_next$total > 30000 & df.purchase_next$total <= 100000,]$class <- "B"
df.purchase_next[df.purchase_next$total > 100000,]$class <- "A"
#新規登録ユーザIDを特定
df.newuserid <- df.dau_now %>%
filter(start_time >= set.now_firstday)
#必要なカラムのみ抽出
#dau
df.dau_before <- df.dau_before %>%
dplyr::select(user_id, playtime)
df.dau_now <- df.dau_now %>%
dplyr::select(user_id, playtime)
df.dau_next <- df.dau_next %>%
dplyr::select(user_id)
df.purchase_now <- df.purchase_now %>%
dplyr::select(user_id,class)
df.purchase_next <- df.purchase_next %>%
dplyr::select(user_id,class)
#既存ユーザ属性特定
#join to dau
df.all_now <- left_join(df.dau_now,df.purchase_now,by = "user_id")
df.all_now[is.na(df.all_now)] <- "NP"
#離脱ユーザの特定と新規除外
#z:before > now
df.all_now_old <- left_join(df.dau_before,df.all_now, by = "user_id")
df.all_now_old[is.na(df.all_now_old)] <- "Z"
#np:now > next
df.all_next <- left_join(df.dau_next,df.purchase_next,by = "user_id")
df.all_next[is.na(df.all_next)] <- "NP"
#z:now > next
df.all_now_old <- left_join(df.all_now_old, df.all_next, by = "user_id")
df.all_now_old[is.na(df.all_now_old)] <- "Z"
#rename and select
df.all_old <- df.all_now_old %>%
dplyr::select(user_id, playtime.x, class.x, class.y)
names(df.all_old) <- c("user_id","playtime","class_now","class_next")
#新規ユーザ属性特定
df.dau_now_new <- left_join(df.newuserid, df.dau_now, by = "user_id")
df.all_now_new <- left_join(df.dau_now_new, df.purchase_now, by = "user_id")
df.all_now_new[is.na(df.all_now_new)] <- "NP"
#z:now > next
df.all_now_new <- left_join(df.all_now_new, df.all_next, by = "user_id")
df.all_now_new[is.na(df.all_now_new)] <- "Z"
#rename and select
df.all_new <- df.all_now_new %>%
dplyr::select(user_id, playtime.x, class.x, class.y)
names(df.all_new) <- c("user_id","playtime","class_now","class_next")
#新規をrbind
df.all <- rbind(df.all_new, df.all_old)
#決定木モデル構築
#playtimは変数として使うとシミュレーションが困難となるため使用しない
tree <- rpart(class_next ~ class_now,data = df.all,control=rpart.control(minsplit=1, cp=0.0001))
dev.off() #エラー回避→ Error in .Call.graphics(C_palette2, .Call(C_palette2, NULL)) :invalid graphics state
plot(as.party(tree))
plotcp(tree)
#遷移確率
class_now <- df.all$class_now
df.test <- data.frame(class_now)
df.pred <- predict(tree,df.test)
df.pred <- data.frame(df.pred)
df.pred <- cbind(df.test,df.pred) #横に連結
#write.csv(df.pred,"./output/pred.csv")
#確率
df.pred_Pv <- melt(df.pred, id.vars = c("class_now"),variable.name = "class_next", na.rm = TRUE)
df.pred_Pv <- df.pred_Pv %>%
group_by(class_now,class_next) %>%
summarise(Pv = mean(value))
write.csv(df.pred_Pv,"./output/pred_Pv.csv")
#初期化 ↓
#initial data
df.initialdata <- df.all_now
df.initialdata <- df.initialdata %>%
group_by(playtime, class) %>%
summarise(uu = length(user_id))
names(df.initialdata) <- c("playtime","class_now","uu")
#sum = a~npの合計
anp_before <- sum(df.initialdata$uu)
#newuser
df.newuser_PayPv <- left_join(df.dau_now,df.purchase_now,by = "user_id")
df.newuser_PayPv <- df.newuser_PayPv %>%
filter(playtime <= 30) #1ヶ月は30日とする
df.newuser_PayPv[is.na(df.newuser_PayPv)] <- "NP"
#newuser Pv
df.newuser_PayPv <- df.newuser_PayPv %>%
group_by(class) %>%
summarize(uu = length(user_id))
df.newuser_PayPv$Pv <- df.newuser_PayPv$uu / sum(df.newuser_PayPv$uu)
#設定
df.newuser_PayPv$uu <- df.newuser_PayPv$Pv * newusers
df.newuser_PayPv$playtime <- 1
df.newuser_PayPv <- df.newuser_PayPv %>%
group_by(playtime, class) %>%
summarise(uu = sum(uu))
names(df.newuser_PayPv) <- c("playtime","class_now","uu")
df.result <- df.initialdata
df.result$turn <- 0
#simulation 最初の予測
#変数データを用意 playtime class_now
df.sim <- left_join(df.initialdata, df.pred_Pv, by = "class_now")
df.sim$uu_next <- df.sim$uu * df.sim$Pv
df.sim <- df.sim %>%
group_by(playtime, class_next) %>%
summarise(uu = sum(uu_next))
names(df.sim) <- c("playtime","class_now","uu")
df.sim$playtime <- df.sim$playtime + 30 #1ヶ月は30日とする
#次の新規挿入
df.sim <- rbind(df.newuser_PayPv,df.sim)
#前月actZrate
df.sim[df.sim$class_now == "Z",]$uu <- df.sim[df.sim$class_now == "Z",]$uu * ActZrate
#初期値に代入
df.initialdata <- df.sim
df.result_test <- df.initialdata
df.result_test$turn <- 1
df.result <- rbind(df.result, df.result_test)
#simulation 2回目以降の予測
#初期化
#予測
for(i in 2:30){ #30ヵ月後までシミュレート(任意)
#変数データを用意 playtime class_now
df.sim <- left_join(df.initialdata, df.pred_Pv, by = "class_now")
df.sim$uu_next <- df.sim$uu * df.sim$Pv
df.sim <- df.sim %>%
group_by(playtime, class_next) %>%
summarise(uu = sum(uu_next))
names(df.sim) <- c("playtime","class_now","uu")
df.sim$playtime <- df.sim$playtime + 30 #1ヶ月は30日とする
#前月actZrate
df.sim[df.sim$class_now == "Z",]$uu <- df.sim[df.sim$class_now == "Z",]$uu * ActZrate
#次の新規挿入
df.sim <- rbind(df.newuser_PayPv,df.sim)
#初期値に代入
df.initialdata <- df.sim
df.result_test <- df.initialdata
df.result_test$turn <- i
df.result <- rbind(df.result, df.result_test)
}
#結果出力
write.csv(df.result,"./output/result.csv")
#遷移確率のネットワーク図
df.Pv_netwaork <- acast(df.pred_Pv, class_now ~ class_next, max, value.var = "Pv")
gplot(df.Pv_netwaork,mode = "circle",edge.lwd = df.Pv_netwaork*15, edge.col = "#c8c8cb", displaylabels = TRUE)
【統計】 二項分布の期待値と分散
確率:p
試行回数:n
二項分布の期待値
E(X) = np
二項分布の分散
V(X) = np(1-p)
・参照
二項分布の平均と分散の二通りの証明
http://mathtrain.jp/bin
二項分布 wikipedia
https://ja.wikipedia.org/wiki/%E4%BA%8C%E9%A0%85%E5%88%86%E5%B8%83
試行回数:n
二項分布の期待値
E(X) = np
二項分布の分散
V(X) = np(1-p)
・参照
二項分布の平均と分散の二通りの証明
http://mathtrain.jp/bin
二項分布 wikipedia
https://ja.wikipedia.org/wiki/%E4%BA%8C%E9%A0%85%E5%88%86%E5%B8%83
2016年5月18日水曜日
【おもしろ動画】オリエンタルラジオ 『PERFECT HUMAN』 ENGEI 2016.2.13
僕はめっちゃ笑いましたよ
(めっちゃ良い分析できた!あいむあぱーふぇくとひゅーまん!)
歌ネタもおもしろけば良い!
パーフェクトヒューマン!
(めっちゃ良い分析できた!あいむあぱーふぇくとひゅーまん!)
歌ネタもおもしろけば良い!
パーフェクトヒューマン!
2016年5月16日月曜日
2016年5月10日火曜日
【Google Spreadsheet】200万セル制限を回避
手順
http://hacknote.jp/archives/16575/
- インポート元のマスターデータを管理するスプレッドシートを用意
- FILTER 関数を利用して、行のフィルタリングを実施。列のフィルタリングを行う場合は ARRAYFORMULA 関数を利用して必要な列をピックアップ
- 不要な空列は事前に削除
- 1.とは別の、閲覧用のスプレッドシートを用意
- IMPORTRANGE 関数を利用して、マスターデータのスプレッドシートからデータを抽出
http://hacknote.jp/archives/16575/
登録:
投稿
(
Atom
)